Exercise with Fractals
Last updated on 2023-01-11 | Edit this page
Estimated time: 60 minutes
Overview
Questions
- Can we try a real problem now?
Objectives
- Create a strategy to parallelise existing code
- Apply previous lessons
The Mandelbrot and Julia fractals
This exercise uses Numpy and Matplotlib.
We will be computing the famous Mandelbrot fractal.
Complex numbers
Complex numbers are a special representation of rotations and scalings in the two-dimensional plane. Multiplying two complex numbers is the same as taking a point, rotate it by an angle \(\phi\) and scale it by the absolute value. Multiplying with a number \(z \in \mathbb{C}\) by 1 preserves \(z\). Multiplying a point at \(i = (0, 1)\) (having a positive angle of 90 degrees and absolute value 1), rotates it anti-clockwise by 90 degrees. Then you might see that \(i^2 = (-1, 0)\). The funny thing is, that we can treat \(i\) as any ordinary number, and all our algebra still works out. This is actually nothing short of a miracle! We can write a complex number
\[z = x + iy,\]
remember that \(i^2 = -1\) and act as if everything is normal!
The Mandelbrot set is the set of complex numbers \[c \in \mathbb{C}\] for which the iteration,
\[z_{n+1} = z_n^2 + c,\]
converges, starting iteration at \(z_0 = 0\). We can visualize the Mandelbrot set by plotting the number of iterations needed for the absolute value \(|z_n|\) to exceed 2 (for which it can be shown that the iteration always diverges).
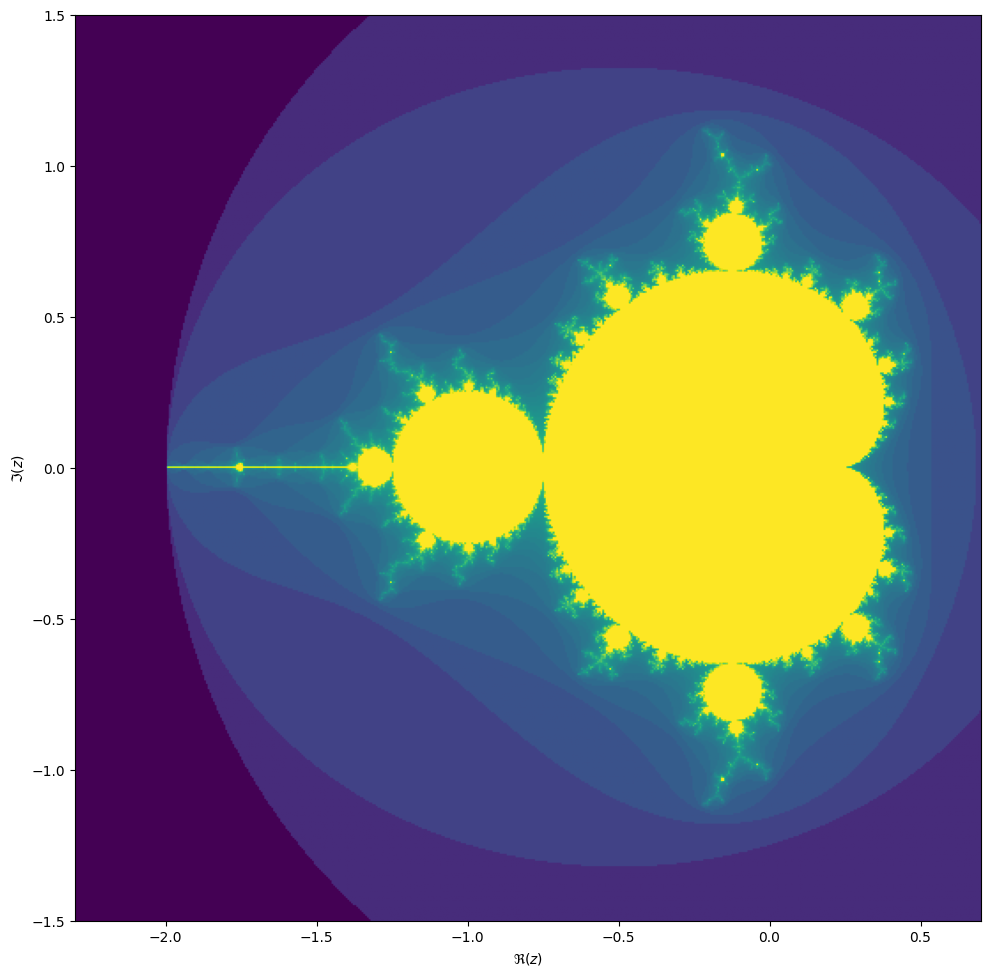
We may compute the Mandelbrot as follows:
PYTHON
max_iter = 256
width = 256
height = 256
center = -0.8+0.0j
extent = 3.0+3.0j
scale = max((extent / width).real, (extent / height).imag)
result = np.zeros((height, width), int)
for j in range(height):
for i in range(width):
c = center + (i - width // 2 + (j - height // 2)*1j) * scale
z = 0
for k in range(max_iter):
z = z**2 + c
if (z * z.conjugate()).real > 4.0:
break
result[j, i] = k
Then we can plot with the following code:
PYTHON
fig, ax = plt.subplots(1, 1, figsize=(10, 10))
plot_extent = (width + 1j * height) * scale
z1 = center - plot_extent / 2
z2 = z1 + plot_extent
ax.imshow(result**(1/3), origin='lower', extent=(z1.real, z2.real, z1.imag, z2.imag))
ax.set_xlabel("$\Re(c)$")
ax.set_ylabel("$\Im(c)$")
Things become really loads of fun when we start to zoom in. We can
play around with the center
and extent
values
(and necessarily max_iter
) to control our window.
When we zoom in on the Mandelbrot fractal, we get smaller copies of the larger set!
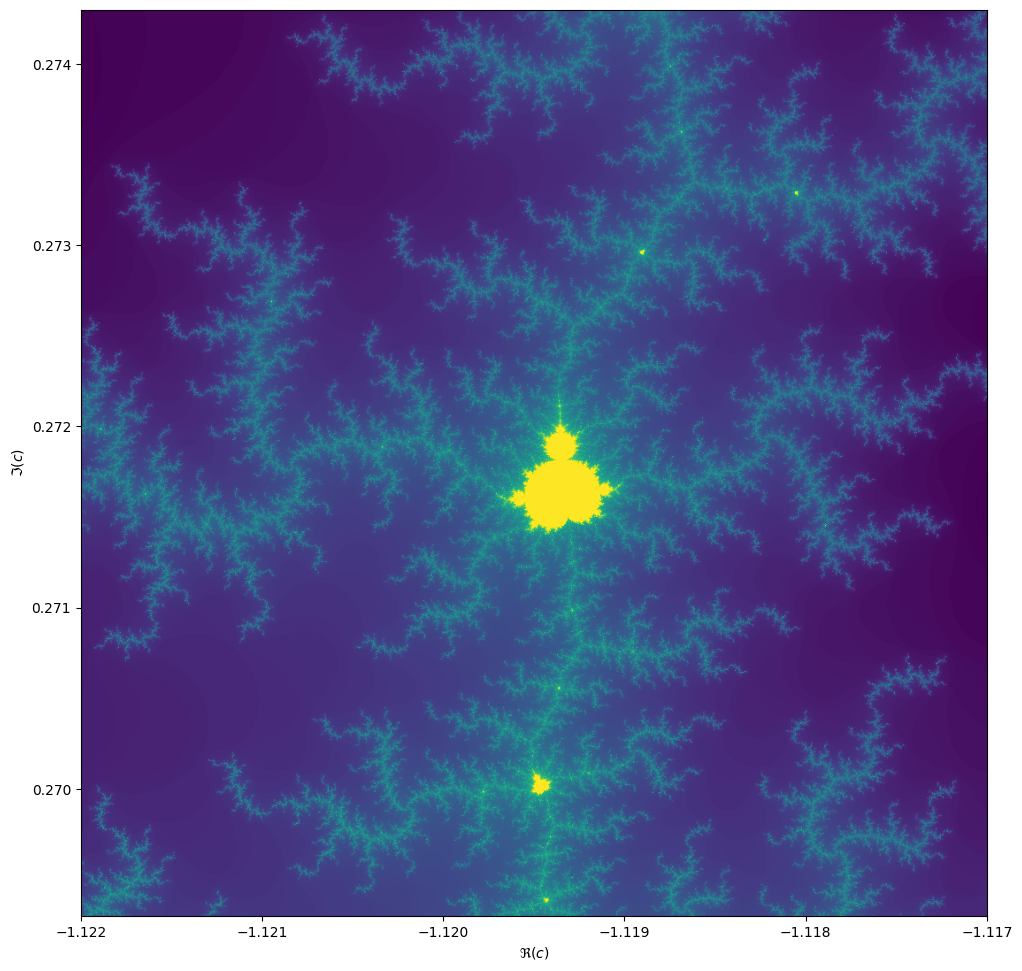
Exercise
Make this into an efficient parallel program. What kind of speed-ups do you get?
We start with a naive implementation. It may be convenient to define
a BoundingBox
class in a separate module
bounding_box.py
. We’ll add methods to this class later
on.
PYTHON
from dataclasses import dataclass
from typing import Optional
import numpy as np
import dask.array as da
@dataclass
class BoundingBox:
width: int
height: int
center: complex
extent: complex
_scale: Optional[float] = None
@property
def scale(self):
if self._scale is None:
self._scale = max(self.extent.real / self.width,
self.extent.imag / self.height)
return self._scale
<<bounding-box-methods>>
test_case = BoundingBox(1024, 1024, -1.1195+0.2718j, 0.005+0.005j)
PYTHON
import matplotlib # type:ignore
matplotlib.use(backend="Agg")
from matplotlib import pyplot as plt
import numpy as np
from .bounding_box import BoundingBox
def plot_fractal(box: BoundingBox, values: np.ndarray, ax=None):
if ax is None:
fig, ax = plt.subplots(1, 1, figsize=(10, 10))
else:
fig = None
plot_extent = (box.width + 1j * box.height) * box.scale
z1 = box.center - plot_extent / 2
z2 = z1 + plot_extent
ax.imshow(values, origin='lower', extent=(z1.real, z2.real, z1.imag, z2.imag),
cmap=matplotlib.colormaps["jet"])
ax.set_xlabel("$\Re(c)$")
ax.set_ylabel("$\Im(c)$")
return fig, ax
The main approach with Python will be: use Numba to make this fast.
Then there are two ways to parallelize: let Numba parallelize the
function, or do a manual domain decomposition and use one of many ways
in Python to run things multi-threaded. There is a third way: create a
vectorized function and parallelize using dask.array
. This
last option is almost always slower than
@njit(parallel=True)
or domain decomposition.
PYTHON
When we port the core Mandelbrot function to Numba, we need to keep some best practices in mind:
- Don’t pass composite objects other than Numpy arrays.
- Avoid acquiring memory inside a Numba function; create an array in Python, then pass it to the Numba function.
- Write a Pythonic wrapper around the Numba function for easy use.
PYTHON
from typing import Any, Optional
import numba # type:ignore
import numpy as np
from .bounding_box import BoundingBox
@numba.njit(nogil=True)
def compute_mandelbrot_numba(
result, width: int, height: int, center: complex,
scale: complex, max_iter: int):
for j in range(height):
for i in range(width):
c = center + (i - width // 2 + (j - height // 2) * 1j) * scale
z = 0.0+0.0j
for k in range(max_iter):
z = z**2 + c
if (z*z.conjugate()).real >= 4.0:
break
result[j, i] = k
return result
def compute_mandelbrot(
box: BoundingBox, max_iter: int,
result: Optional[np.ndarray[np.int64]] = None,
throttle: Any = None):
result = result if result is not None \
else np.zeros((box.height, box.width), np.int64)
return compute_mandelbrot_numba(
result, box.width, box.height, box.center, box.scale,
max_iter=max_iter)
Numba parallel=True
We can parallelize loops directly with Numba. Pass the flag
parallel=True
and use prange
to create the
loop. Here it is even more important to obtain the result array outside
the context of Numba, or the result will be slower than the serial
version.
PYTHON
from typing import Optional
import numba # type:ignore
from numba import prange # type:ignore
import numpy as np
from .bounding_box import BoundingBox
@numba.njit(nogil=True, parallel=True)
def compute_mandelbrot_numba(
result, width: int, height: int, center: complex, scale: complex,
max_iter: int):
for j in prange(height):
for i in prange(width):
c = center + (i - width // 2 + (j - height // 2) * 1j) * scale
z = 0.0+0.0j
for k in range(max_iter):
z = z**2 + c
if (z*z.conjugate()).real >= 4.0:
break
result[j, i] = k
return result
def compute_mandelbrot(box: BoundingBox, max_iter: int,
throttle: Optional[int] = None):
if throttle is not None:
numba.set_num_threads(throttle)
result = np.zeros((box.height, box.width), np.int64)
return compute_mandelbrot_numba(
result, box.width, box.height, box.center, box.scale,
max_iter=max_iter)
We split the computation into a set of sub-domains. The
BoundingBox.split()
method is designed such that if we
deep-map the resulting list-of-lists, we can recombine the results using
numpy.block()
.
PYTHON
def split(self, n):
"""Split the domain in nxn subdomains, and return a grid of BoundingBoxes."""
w = self.width // n
h = self.height // n
e = self.scale * w + self.scale * h * 1j
x0 = self.center - e * (n / 2 - 0.5)
return [[BoundingBox(w, h, x0 + i * e.real + j * e.imag * 1j, e)
for i in range(n)]
for j in range(n)]
To perform the computation in parallel, lets go ahead and chose the
most difficult path: asyncio
. There are other ways to do
this, setting up a number of threads, or use Dask. However,
asyncio
is available to us in Python natively. In the end,
the result is very similar to what we would get using
dask.delayed
.
This may seem as a lot of code, but remember: we only used Numba to compile the core part and then used Asyncio to parallelize. The progress bar is a bit of flutter and the semaphore is only there to throttle the computation to fewer cores. Even then, this solution is by far the most extensive, but also the fastest.
PYTHON
from typing import Optional
import numpy as np
import asyncio
from psutil import cpu_count # type:ignore
from contextlib import nullcontext
from .bounding_box import BoundingBox
from .numba_serial import compute_mandelbrot as mandelbrot_serial
async def a_compute_mandelbrot(
box: BoundingBox,
max_iter: int,
semaphore: Optional[asyncio.Semaphore]):
async with semaphore or nullcontext():
result = np.zeros((box.height, box.width), np.int64)
await asyncio.to_thread(
mandelbrot_serial, box, max_iter, result=result)
return result
async def a_domain_split(box: BoundingBox, max_iter: int,
sem: Optional[asyncio.Semaphore]):
n_cpus = cpu_count(logical=True)
split = box.split(n_cpus)
split_result = await asyncio.gather(
*(asyncio.gather(
*(a_compute_mandelbrot(b, max_iter, sem)
for b in row))
for row in split))
return np.block(split_result)
def compute_mandelbrot(box: BoundingBox, max_iter: int,
throttle: Optional[int] = None):
sem = asyncio.Semaphore(throttle) if throttle is not None else None
return asyncio.run(a_domain_split(box, max_iter, sem))
Another solution is to use Numba’s @guvectorize
decorator. The speed-up (on my machine) is not as dramatic as with the
domain-decomposition though.
PYTHON
def grid(self):
"""Return the complex values on the grid in a 2d array."""
x0 = self.center - self.extent / 2
x1 = self.center + self.extent / 2
g = np.mgrid[x0.imag:x1.imag:self.height*1j,
x0.real:x1.real:self.width*1j]
return g[1] + g[0]*1j
def da_grid(self):
"""Return the complex values on the grid in a 2d array."""
x0 = self.center - self.extent / 2
x1 = self.center + self.extent / 2
x = np.linspace(x0.real, x1.real, self.width, endpoint=False)
y = np.linspace(x0.imag, x1.imag, self.height, endpoint=False)
g = da.meshgrid(x, y)
return g[1] + g[0]*1j
PYTHON
from typing import Any
from numba import guvectorize, int64, complex128 # type:ignore
import numpy as np
from .bounding_box import BoundingBox
@guvectorize([(complex128[:, :], int64, int64[:, :])],
"(n,m),()->(n,m)",
nopython=True)
def compute_mandelbrot_numba(inp, max_iter: int, result):
for j in range(inp.shape[0]):
for i in range(inp.shape[1]):
c = inp[j, i]
z = 0.0+0.0j
for k in range(max_iter):
z = z**2 + c
if (z*z.conjugate()).real >= 4.0:
break
result[j, i] = k
def compute_mandelbrot(box: BoundingBox, max_iter: int, throttle: Any = None):
result = np.zeros((box.height, box.width), np.int64)
c = box.grid()
compute_mandelbrot_numba(c, max_iter, result)
return result
PYTHON
from typing import Optional
import timeit
from . import numba_serial, numba_parallel, vectorized, domain_splitting
from .bounding_box import BoundingBox, test_case
compile_box = BoundingBox(16, 16, 0.0+0.0j, 1.0+1.0j)
timing_box = test_case
def compile_run(m):
m.compute_mandelbrot(compile_box, 1)
def timing_run(m, throttle: Optional[int] = None):
m.compute_mandelbrot(timing_box, 1024, throttle=throttle)
modules = ["numba_serial:1", "vectorized:1"] \
+ [f"domain_splitting:{n}" for n in range(1, 9)] \
+ [f"numba_parallel:{n}" for n in range(1, 9)]
if __name__ == "__main__":
with open("timings.txt", "w") as out:
headings = ["name", "n", "min", "mean", "max"]
print(f"{headings[0]:<20}" \
f"{headings[1]:>10}" \
f"{headings[2]:>10}" \
f"{headings[3]:>10}" \
f"{headings[4]:>10}",
file=out)
for mn in modules:
m, n = mn.split(":")
n_cpus = int(n)
setup = f"from mandelbrot.bench_all import timing_run, compile_run\n" \
f"from mandelbrot import {m}\n" \
f"compile_run({m})"
times = timeit.repeat(
stmt=f"timing_run({m}, {n_cpus})",
setup=setup,
number=1,
repeat=50)
print(f"{m:20}" \
f"{n_cpus:>10}" \
f"{min(times):10.5g}" \
f"{sum(times)/len(times):10.5g}" \
f"{max(times):10.5g}",
file=out)
import pandas as pd
from plotnine import ggplot, geom_point, geom_ribbon, geom_line, aes
timings = pd.read_table("timings.txt", delimiter=" +", engine="python")
plot = ggplot(timings, aes(x="n", y="mean", ymin="min", ymax="max",
color="name", fill="name")) \
+ geom_ribbon(alpha=0.3, color="none") \
+ geom_point() + geom_line()
plot.save("mandelbrot-timings.svg")
Extra: Julia sets
For each value \[c\] we can compute the Julia set, namely the set of starting values \[z_1\] for which the iteration over \[z_{n+1}=z_n^2 + c\] converges. Every location on the Mandelbrot image corresponds to its own unique Julia set.
PYTHON
max_iter = 256
center = 0.0+0.0j
extent = 4.0+3.0j
scale = max((extent / width).real, (extent / height).imag)
result = np.zeros((height, width), int)
c = -1.1193+0.2718j
for j in range(height):
for i in range(width):
z = center + (i - width // 2 + (j - height // 2)*1j) * scale
for k in range(max_iter):
z = z**2 + c
if (z * z.conjugate()).real > 4.0:
break
result[j, i] = k
If we take the center of the last image, we get the following rendering of the Julia set:
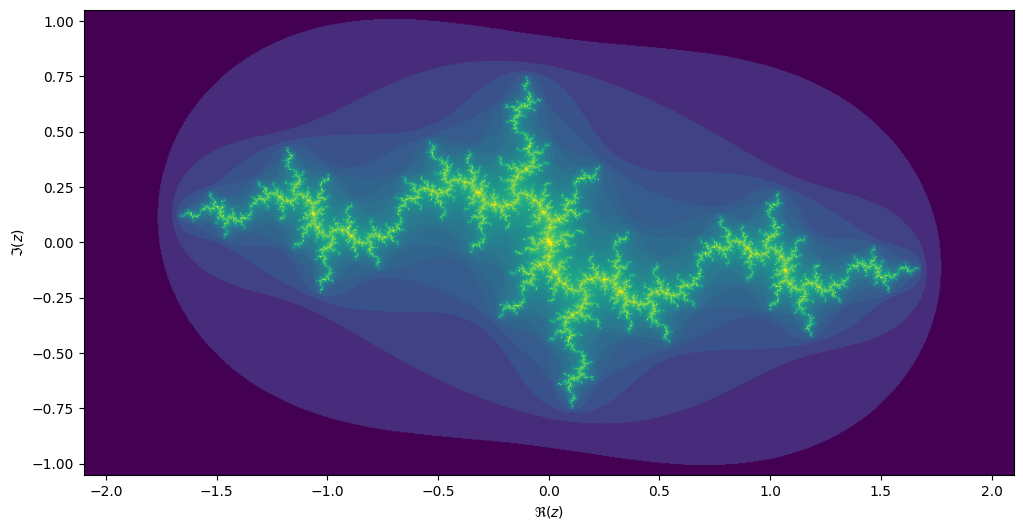
Generalize
Can you generalize your Mandelbrot code, such that you can compute both the Mandelbrot and the Julia sets in an efficient manner, while reusing as much of the code?
Key Points
- Actually making code faster is not always straight forward
- Easy one-liners can get you 80% of the way
- Writing clean, modular code often makes it easier to parallelise later on